Top 10 Data Analysis Tools in 2024: Boost Your Insights
DATA ANALYSIS
Aug 31, 2024

As we move further into the digital age, data is becoming more important than ever. Businesses need to understand and use data to stay ahead. Data analysis tools help make sense of all this information. These tools help businesses make better decisions and improve their performance. In this article, we'll look at the top 10 data analysis tools for 2024. These tools will help you get the most out of your data.
Among these tools, newcomers like MageMetrics are revolutionizing the field with AI-powered data companions, offering a glimpse into the future of data analysis.
Key Takeaways
Data analysis tools are essential for making sense of large amounts of data.
These tools help businesses make better decisions based on data insights.
Each tool has unique features that cater to different data analysis needs.
Using the right tool can improve business efficiency and profits.
Staying updated with the latest tools is crucial for staying competitive.
AI-powered tools like MageMetrics are changing the landscape by making complex data analysis accessible to non-technical users.
MageMetrics
MageMetrics is revolutionizing the data analysis landscape with its AI-powered data companion. This innovative platform is designed to transform your data experience, making it an essential tool for businesses looking to harness the power of their data without getting bogged down in the technical details.
Key Features
AI-Powered Assistant: An intelligent companion that understands your goals and guides you through complex analyses.
No-Code Platform: Intuitive interface that allows users to perform sophisticated data analysis without coding skills.
Real-Time Insights: Transforms raw data into clear, actionable insights instantly.
Automated Data Cleaning: AI assistant handles the tedious task of cleaning and preparing messy data.
Trend Highlighting: Automatically identifies and highlights key trends in your data.
Cross-Source Integration: Seamlessly pulls together data from various sources, including sales reports and other business metrics.
Natural Language Interaction: Communicate with your data using everyday language, making complex queries simple.
Pricing
MageMetrics is currently offering an exclusive 12-month private beta program for select companies. This program includes:
Lifetime discount on future licenses and plans after the first year
Strategic collaboration to influence feature development and product roadmap
Increased internal expertise through direct collaboration with MageMetrics' expert engineers and founders
Participation in the program requires:
Provision of raw upstream data (CRM, ERP, etc.) for analysis
Specification of related downstream analytics goals, KPIs, or metrics
Monthly feedback sessions
A minimal fee to cover infrastructure costs and support program operations
For specific pricing details and to join the private beta program, interested companies can visit the MageMetrics website or contact them directly.
Pros and Cons
Pros:
Dramatically reduces time spent on data wrangling
Makes advanced data analysis accessible to non-technical users
Provides actionable insights in real-time
Improves decision-making efficiency
Continuously learns and adapts to your specific business needs
Cons:
Currently in private beta, so not widely available yet
May require initial setup and integration with existing systems
Tableau
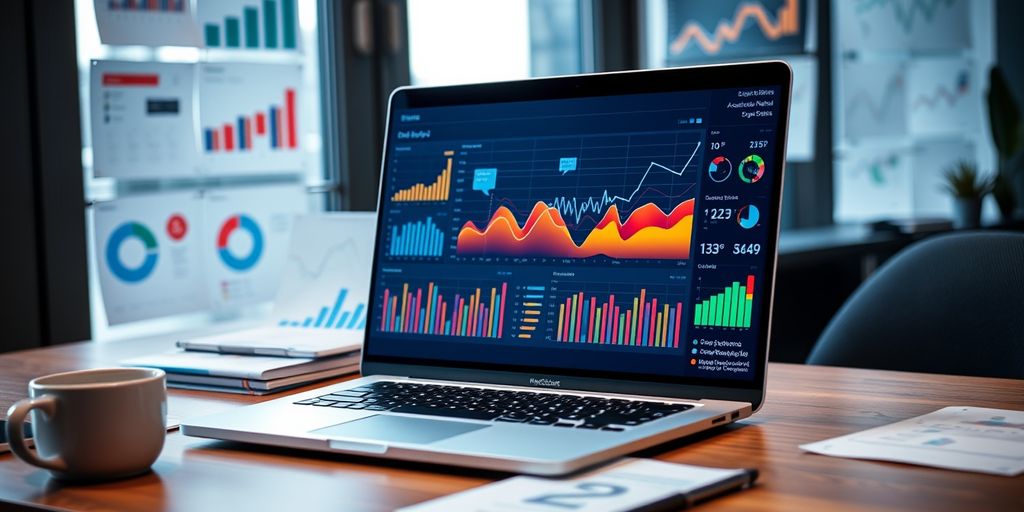
Tableau is a user-friendly data analytics tool known for its drag-and-drop interface. This feature allows users to create interactive visuals and dashboards effortlessly. Organizations can quickly turn raw data into meaningful visuals, making it easy to understand.
Key Features
Easy Drag and Drop Interface
Mobile support for both iOS and Android
Data Discovery feature to find hidden data
Supports various data sources like SQL Server and Oracle
Tableau's strength lies in its quality visuals embedded with interactive information, making it a top choice for harnessing business intelligence and big data for competitive advantage.
However, Tableau is not without its drawbacks. It is primarily designed for data visualization, so it lacks data preprocessing capabilities. Additionally, it has a learning curve and can be expensive.
Pros & Cons
Pros:
Offers a free trial
Backed by Salesforce
In-person and virtual conferences
Cons:
No scheduling of reports
High cost for enterprise solutions
Tableau is ideal for businesses looking to gain insights from their data through powerful visualizations. Despite its cost and learning curve, its capabilities make it a valuable tool for data analysis.
Power BI
Power BI is a cloud-based business analytics tool from Microsoft. It lets you combine data from different sources, analyze it, and present it through visualizations, reports, and dashboards. Power BI is known for its excellent data connectivity, working seamlessly with Excel, text files, SQL servers, and cloud sources like Google and Facebook analytics.
Key Features
Direct connectivity to Excel and easy integration with other Microsoft products
Ability to compress data and extract insights from large datasets
Customizability using R and Python
Using Power Query to import, filter, and translate data
An adaptive and friendly user experience
Pros and Cons
Pros:
Mobile app
Offers a free trial
Easy to use, especially if you already know Excel
Cons:
Too much data can slow it down
Data limits may require you to upgrade plans
Power BI is essential for businesses aiming to thrive in a competitive landscape. It provides real-time insights and predictive analytics, enabling companies to make data-driven decisions, optimize resources, and enhance financial performance.
Apache Spark
Apache Spark is a powerful data processing framework that is open-source and widely used for big data processing and machine learning. It was first developed in 2012 and is designed to analyze unstructured big data by distributing computationally heavy tasks across many computers.
Key Features
Speed and Efficiency: Spark is known for its incredible speed, thanks to its in-memory processing capabilities, making it around 100x faster than Hadoop.
Machine Learning Library (MLlib): Includes a variety of algorithms for classification, regression, and clustering.
Integration: Works well with other languages and systems, including Python, Scala, R, and SQL shells.
Versatility: Can run on various platforms like Hadoop, Kubernetes, Cloud, and standalone.
Use Cases
Big Data Processing: Ideal for handling and manipulating large datasets in real-time.
Machine Learning: Often used for developing data-heavy machine learning models.
Apache Spark is a go-to tool for data analysts and scientists aiming to enhance efficiency and competitiveness through automated data processes.
While it has some drawbacks, such as lacking a file management system and being computationally expensive, its speed and versatility make it a top choice for many data professionals.
Hadoop
Hadoop by Apache is a powerful tool for big data analytics. It is an open-source framework that helps store and process large amounts of data using the MapReduce model. This makes it a popular choice among professionals.
Key Features
Open Source: Free to use and customize.
Scalability: Can handle large data sets by distributing them across multiple nodes.
Fault Tolerance: Continues to operate even if some nodes fail.
Commodity Hardware: Can run on less expensive hardware.
Advantages
Cost-Effective: Since it runs on commodity hardware, it reduces costs.
Flexibility: Supports various data formats and can be customized.
Reliability: Fault-tolerant design ensures data processing continues even during failures.
Disadvantages
Learning Curve: Hadoop can be hard to learn, especially the MapReduce model.
Hardware Requirements: Needs powerful hardware to run efficiently.
Hadoop is a cornerstone in the world of big data, offering a reliable and scalable solution for data storage and processing.
R
R is a powerful open-source programming language widely used for statistical computing and data analysis. It is known for its ability to handle large datasets and its flexibility in various areas like data visualization and data processing. R's built-in graphics capabilities allow users to create stunning visuals, making it a favorite among data scientists.
Features
Ability to handle large datasets
Flexibility in data visualization and processing
Built-in graphics capabilities
Active community support
R is a great choice for those who need to clean, manage, and monitor data effectively. Its extensive package library includes tools for data cleaning, machine learning, and natural language processing, making it a versatile tool for data analysis.
Despite its many strengths, R does have some drawbacks. It is significantly slower than languages like C++ and Java and has a steep learning curve, especially for those unfamiliar with programming. However, the active community and the availability of numerous packages make it easier to overcome these challenges.
Python & R
Python is a programming language with a wide range of uses, making it essential for any data analyst. Unlike more complex languages, it focuses on readability, and its general popularity in the tech field means many programmers are already familiar with it.
Python is also extremely versatile; it has a huge range of resource libraries suited to a variety of different data analytics tasks. For example, the NumPy and pandas libraries are great for streamlining highly computational tasks, as well as supporting general data manipulation.
Libraries like Beautiful Soup and Scrapy are used to scrape data from the web, while Matplotlib is excellent for data visualization and reporting. Python’s main drawback is its speed—it is memory intensive and slower than many languages. In general though, if you’re building software from scratch, Python’s benefits far outweigh its drawbacks. You can learn more about Python in our full guide.
R, like Python, is a popular open-source programming language. It is commonly used to create statistical/data analysis software.
R at a glance:
Type of tool: Programming language.
Availability: Open-source.
Mostly used for: Statistical analysis and data mining.
Pros: Platform independent, highly compatible, lots of packages.
Cons: Slower, less secure, and more complex to learn than Python.
R’s syntax is more complex than Python and the learning curve is steeper. However, it was built specifically to deal with heavy statistical computing tasks and is very popular for data visualization. A bit like Python, R also has a network of freely available code, called CRAN (the Comprehensive R Archive Network), which offers 10,000+ packages.
SAS
SAS, which stands for Statistical Analysis System, is a powerful tool widely used for business analytics. Developed by the SAS Institute, it offers both a graphical user interface and a terminal interface, making it versatile for users with different skill levels. SAS is known for its robust analytics and can handle large datasets efficiently.
Key Features
Robust Analytics: Offers a wide range of advanced analytical tools.
Integration: Seamlessly integrates with various data sources and SAS products.
User Interfaces: Provides both graphical and terminal interfaces.
Large Datasets: Capable of handling large datasets effectively.
SAS is a commercial product, which means it comes with a hefty price tag.
Use Cases
SAS is primarily used for:
Business intelligence
Multivariate analysis
Predictive modeling
Customer profiling
Despite its complexity, SAS remains a go-to tool for enterprises needing robust and versatile data analysis solutions.
QlikSense
QlikSense is a powerful business and data analysis tool that supports data visualization and analysis. It integrates with various data sources, including spreadsheets, databases, and cloud services, allowing users to create stunning dashboards and visualizations. QlikSense leverages machine learning and AI to help users understand their data better.
Key Features
Tools for interactive data visualization
Conversational AI-powered analytics with Qlik Insight Bot
Qlik Big Data Index for data indexing
Instant search and natural language processing
Pros & Cons
Pros:
Available via SaaS, the cloud, and on-premises
Integrates with apps like Dropbox, Microsoft Excel, Hive, and Qualtrics
Real-time predictive calculations
Cons:
May require extensive help to set up
No dark mode
Who Should Use QlikSense?
QlikSense is ideal for businesses looking to use machine learning for data analytics. It empowers non-technical users to independently access and analyze data, making it a great choice for organizations aiming to boost data-driven decision-making.
With its high-performance software-as-a-service (SaaS) and hybrid cloud architecture, organizations of all sizes can take advantage of unrivaled analytics performance and versatility.
KNIME
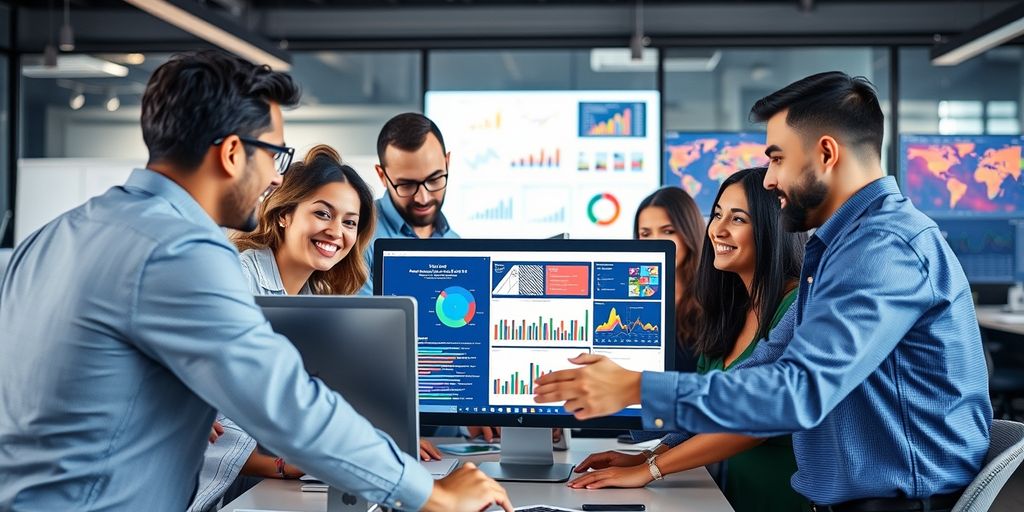
KNIME is a powerful data analysis tool that stands out for its open-source nature and user-friendly interface. Developed initially for the pharmaceutical industry, it has since expanded its reach to various fields, including business intelligence and customer analysis.
One of the key features of KNIME is its drag-and-drop graphical user interface (GUI), which makes it ideal for users with minimal coding knowledge. This intuitive interface allows users to create data workflows effortlessly.
Key Features
Open-source: KNIME is free to use, making it accessible for small businesses with limited budgets.
Data Integration: It supports over 300 data sources, allowing seamless data access and collection.
Extensive Analytics Tools: KNIME offers tools for machine learning, data mining, and big data processing.
Customizable: The platform is highly flexible and can be tailored to meet specific organizational needs.
Conclusion
In 2024, the landscape of data analysis tools is more dynamic and essential than ever. With the right tools, businesses can turn raw data into valuable insights, driving smarter decisions and better outcomes. From user-friendly options like Tableau and Power BI to powerful platforms like Apache Spark and TensorFlow, and innovative AI-driven solutions like MageMetrics, there's a tool for every need and skill level.. As data continues to grow in importance, mastering these tools will be crucial for anyone looking to excel in the field. Whether you're a beginner or a seasoned professional, staying updated with the latest tools will help you stay ahead in the ever-evolving world of data analytics.
Frequently Asked Questions
Why are data analysis tools important?
Data analysis tools help businesses make sense of large amounts of data. They allow companies to make informed decisions, improve efficiency, and increase profits by turning data into valuable insights.
What are the latest trends in data analysis tools?
The latest trend is AI-powered data analysis, with tools like MageMetrics leading the way by offering intelligent assistants that can understand complex queries and provide insights in natural language.
Which data analysis tools are best for beginners?
For beginners, MageMetrics is an excellent choice with its AI-powered assistant that guides users through the analysis process.
What tools are good for handling large datasets?
Tools like MageMetrics, Tableau, Python, R, QlikSense, and SAS are excellent for managing large datasets. They offer powerful features to process and analyze big data effectively.
Can data analysis tools help in decision-making?
Yes, data analysis tools can significantly improve decision-making. They provide insights and trends that help businesses make better and more informed choices.
Are there any free data analysis tools available?
Yes, there are free tools available like Python and R. These tools are open-source and have a large community of users who contribute to their development and provide support.
How do I choose the right data analysis tool for my needs?
Choosing the right tool depends on your specific needs and skill level. Consider what you want to achieve, the size of your datasets, and your familiarity with the tool. Researching and trying out different tools can also help you make an informed decision.