Self-Service Analytics: Empowering Business Teams
BUSINESS INTELLIGENCE
Jul 5, 2024
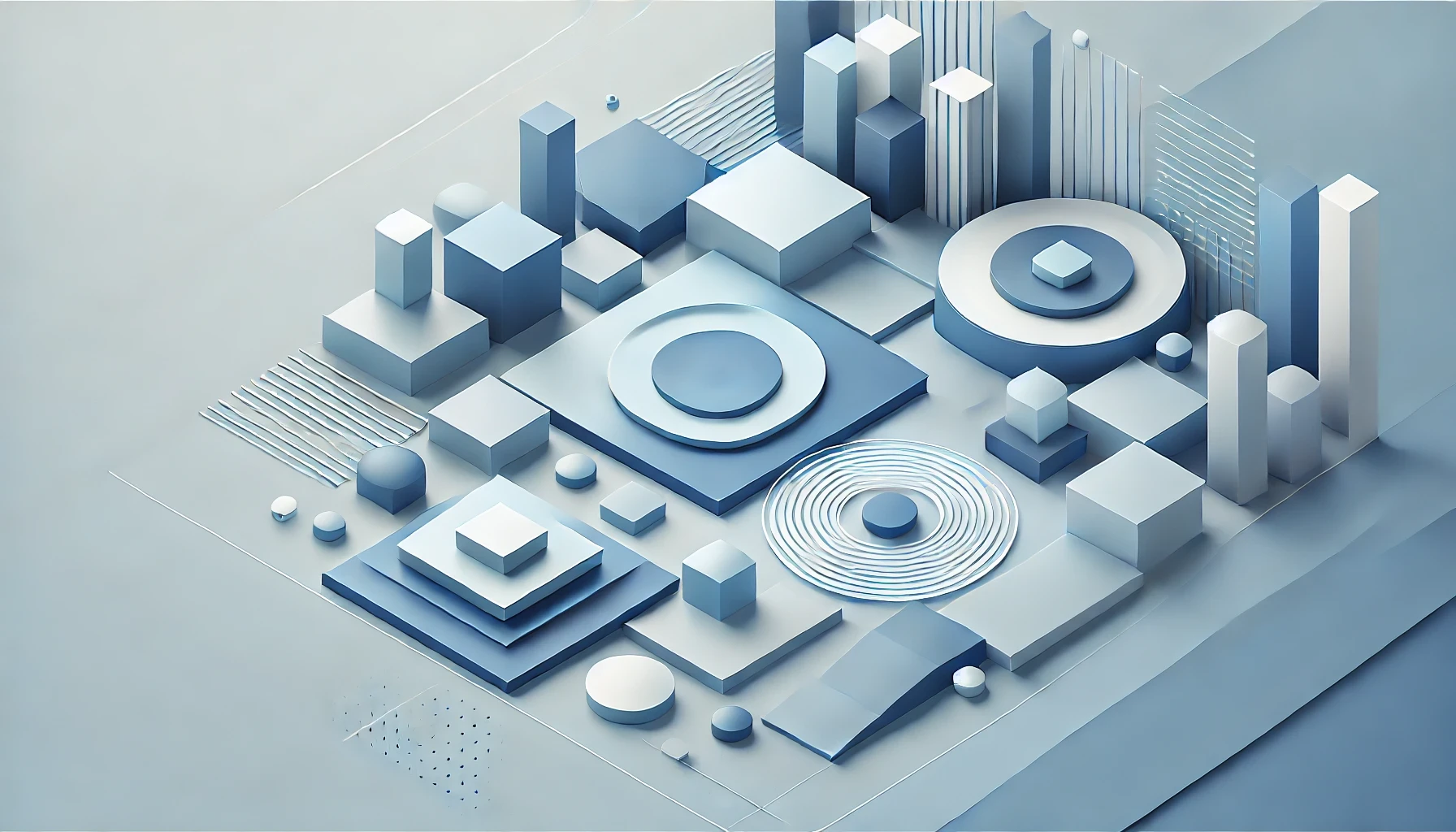
In today's data-driven business landscape, the ability to quickly access and analyze data is crucial for making informed decisions. Self-service analytics has emerged as a powerful tool for empowering business teams to harness the full potential of their data. By enabling non-technical users to explore and visualize data on their own, self-service analytics streamlines decision-making processes, reduces reliance on IT departments, and ultimately drives business growth. In this blog post, we will delve into the concept of self-service analytics, its key features and benefits, strategies for successful implementation, as well as real-world case studies showcasing its transformative impact. Join us as we explore how self-service analytics can revolutionize the way business teams work and collaborate in today's data-driven world.
What is Self-Service Analytics?
Self-service analytics refers to the practice of enabling business users to independently access, analyze, and derive insights from data without the need for extensive technical expertise or reliance on IT departments. It empowers individuals within an organization to explore and interpret data on their own terms, leading to faster decision-making, increased agility, and improved overall efficiency.
Defining Self-Service Analytics
Self-service analytics can be defined as a user-centric approach to data analysis, where individuals have the ability to access and analyze data directly, without requiring assistance from IT professionals or data analysts. It involves the use of intuitive and user-friendly tools that allow users to explore data, create visualizations, generate reports, and gain insights without extensive coding or programming knowledge.
Key Features of Self-Service Analytics
Data Accessibility: Self-service analytics tools provide users with easy access to relevant data sources, including databases, spreadsheets, and cloud storage, allowing them to retrieve and analyze data in real-time.
User-Friendly Interfaces: These tools offer intuitive and user-friendly interfaces that require minimal technical expertise, enabling users to navigate and interact with data effortlessly.
Data Visualization: Self-service analytics allows users to transform raw data into visually appealing and interactive charts, graphs, and dashboards, making it easier to understand and interpret complex information.
Ad Hoc Analysis: With self-service analytics, users can perform ad hoc analysis by applying filters, conducting drill-downs, and creating custom calculations, empowering them to explore data from different angles and uncover hidden insights.
Data Governance and Security: While self-service analytics promotes data accessibility, it also ensures proper governance and security measures to protect sensitive information. It includes features like user permissions, data encryption, and audit trails to maintain data integrity and compliance.
By incorporating these key features, self-service analytics provides business teams with the tools and capabilities to become more self-reliant in their data analysis and decision-making processes. It enables users to go beyond simple reporting and access the information they need, when they need it, leading to greater efficiency and agility within organizations.
Benefits of Self-Service Analytics
Self-service analytics offers numerous benefits to organizations and business teams, empowering them to make data-driven decisions efficiently and effectively. Let's explore some of the key advantages:
Empowering Non-Technical Users
One of the primary benefits of self-service analytics is its ability to empower non-technical users. Traditionally, data analysis and interpretation were limited to IT departments or data analysts with specialized skills. However, self-service analytics tools provide an intuitive and user-friendly interface that enables individuals from various departments to access and analyze data on their own. This empowerment allows business users to directly explore data, generate insights, and make informed decisions without relying on technical experts.
Faster Decision-Making
Self-service analytics significantly accelerates the decision-making process within organizations. With self-service tools, business users can independently access real-time data and generate insights quickly. They no longer need to wait for reports or analysis from IT departments, which can often be time-consuming. By eliminating bottlenecks and reducing dependency, self-service analytics enables users to make faster and more agile decisions, giving organizations a competitive edge in a rapidly changing business environment.
Reduced Dependence on IT
By empowering business users to perform their own data analysis, self-service analytics reduces the burden on IT departments. Instead of relying on IT professionals to generate reports or conduct complex analysis, business teams can independently access and analyze data whenever needed. This frees up IT resources to focus on more strategic and specialized tasks, leading to increased efficiency and productivity across the organization.
Enhanced Collaboration and Communication
Self-service analytics promotes collaboration and communication among team members. With the ability to share visualizations, dashboards, and reports, business users can easily collaborate on data analysis, align their insights, and make collective decisions. This fosters a culture of data-driven collaboration, where teams can leverage the collective knowledge and expertise within the organization to drive innovation and achieve common goals.
Improved Data Quality and Governance
Self-service analytics tools often come equipped with data quality and governance features that ensure the accuracy, consistency, and security of data. By providing users with access to reliable and up-to-date data sources, these tools help maintain data integrity and eliminate the risk of working with outdated or inaccurate information. Additionally, self-service analytics platforms often have built-in data governance capabilities, allowing organizations to enforce data policies, manage access permissions, and ensure compliance with regulatory requirements.
In summary, the benefits of self-service analytics include empowering non-technical users, accelerating decision-making, reducing dependence on IT, enhancing collaboration, and improving data quality and governance. By leveraging these advantages, organizations can unlock the full potential of their data and drive business growth and innovation.
Implementing Self-Service Analytics in Your Organization
Implementing self-service analytics within an organization requires careful planning, consideration of tools and technologies, as well as adequate training and support for the users. Here are some key steps to successfully implement self-service analytics in your organization:
Choosing the Right Tools
The first step in implementing self-service analytics is selecting the appropriate tools and technologies that align with your organization's needs and goals. Consider factors such as ease of use, scalability, integration capabilities, and support for data sources. Some popular self-service analytics tools include Tableau, Power BI, QlikView, and Looker. Evaluate different options, conduct demos, and involve key stakeholders in the decision-making process to ensure the selected tool meets the requirements of your organization.
Defining Data Governance Policies
Establishing data governance policies is crucial to maintain data quality, security, and compliance within a self-service analytics environment. Define data ownership, access controls, and data classification guidelines. Establish clear processes for data validation, data lineage, and change management. It is important to strike a balance between enabling self-service analytics and ensuring data integrity and security.
Training and Support
Training and support are essential to ensure successful adoption of self-service analytics within your organization. Provide comprehensive training programs to familiarize users with the selected tool and its features. Offer hands-on workshops, webinars, and documentation to help users navigate the tool and perform various tasks such as data exploration, visualization, and report generation. Additionally, establish a support system that users can rely on for assistance, troubleshooting, and guidance.
Encouraging Collaboration and Knowledge Sharing
Promote a culture of collaboration and knowledge sharing within your organization to maximize the benefits of self-service analytics. Encourage users to share their experiences, insights, and best practices with others. Implement platforms or forums where users can exchange ideas, ask questions, and seek advice from their peers. Foster cross-functional collaboration by creating communities of practice or organizing regular knowledge-sharing sessions.
Continuous Monitoring and Evaluation
Implementing self-service analytics is an iterative process. Continuously monitor and evaluate the usage, adoption, and impact of self-service analytics within your organization. Gather feedback from users to identify potential challenges and areas for improvement. Regularly review and update data governance policies and training programs based on evolving needs and technology advancements. This ongoing evaluation and improvement process will ensure the long-term success and effectiveness of self-service analytics in your organization.
By following these steps and ensuring a well-planned implementation, your organization can successfully deploy self-service analytics, empowering business teams to access, analyze, and derive insights from data independently, leading to more efficient decision-making and improved business outcomes.
Challenges and Solutions
Implementing self-service analytics in an organization can come with its own set of challenges. However, with proper planning and strategies, these challenges can be overcome. Let's explore some common challenges and their solutions:
Common Challenges
Data Quality and Consistency: One of the key challenges is ensuring the quality and consistency of the data used in self-service analytics. Inaccurate or inconsistent data can lead to incorrect insights and decisions. It is crucial to establish data governance policies, data validation processes, and data cleansing techniques to maintain data integrity.
Data Security and Privacy: Self-service analytics introduces the risk of unauthorized access to sensitive data. Organizations must implement robust security measures, including user access controls, data encryption, and compliance with data protection regulations. Regular security audits and training programs can also help mitigate security risks.
User Adoption and Training: Some users may be resistant to change or lack the necessary skills to effectively use self-service analytics tools. Providing comprehensive training programs, workshops, and ongoing support can help users overcome these challenges and become proficient in using the tools.
Technical Integration and Data Complexity: Integrating self-service analytics tools with existing systems and data sources can be complex. It is essential to ensure compatibility, establish data connections, and address any technical challenges that may arise. Organizations may need to invest in data integration solutions or seek assistance from IT professionals.
Overcoming Resistance to Change
Resistance to change is a common challenge when implementing self-service analytics. Here are some strategies to address this issue:
Communication and Education: Clearly communicate the benefits of self-service analytics to the users. Explain how it can empower them, streamline their workflows, and improve decision-making. Provide educational resources, case studies, and success stories to build trust and enthusiasm among the user community.
Executive Support and Leadership: Obtain support from top-level executives and leaders within the organization. Their endorsement and active participation in the adoption of self-service analytics can help overcome resistance and encourage buy-in from other stakeholders.
Gradual Rollout and Pilot Programs: Consider implementing self-service analytics in a phased manner or starting with pilot programs. This approach allows users to experience the benefits firsthand and provides an opportunity to address any concerns or challenges before scaling the implementation across the organization.
User Involvement and Feedback: Involve users in the decision-making process and seek their input throughout the implementation. Encourage their participation in shaping the self-service analytics initiative, gathering feedback, and incorporating user suggestions. This sense of ownership and involvement can increase acceptance and adoption.
By proactively addressing these challenges and implementing suitable solutions, organizations can overcome obstacles and ensure successful adoption of self-service analytics. This will enable business teams to harness the full potential of their data, drive innovation, and make data-driven decisions with confidence.
Case Studies of Successful Implementations
Real-world examples of successful implementations of self-service analytics can provide valuable insights and inspiration for organizations looking to adopt this powerful tool. Let's explore some case studies that highlight the transformative impact of self-service analytics:
Real-World Examples
Company X: Company X, a global retail chain, implemented self-service analytics to empower its store managers with real-time insights. By providing intuitive analytics tools, store managers were able to access and analyze sales data, inventory levels, and customer trends. This allowed them to make data-driven decisions on product assortments, pricing strategies, and promotional activities. As a result, Company X experienced improved sales performance, reduced excess inventory, and increased customer satisfaction.
Organization Y: Organization Y, a healthcare provider, adopted self-service analytics to enhance its operational efficiency. By enabling non-technical staff to analyze patient data, track resource utilization, and identify bottlenecks in the system, Organization Y was able to optimize workflows, reduce waiting times, and improve patient outcomes. The self-service analytics platform also facilitated collaboration among various departments, leading to better coordination and communication.
Company Z: Company Z, a financial services firm, implemented self-service analytics to empower its financial advisors. By providing them with self-service tools, advisors could access client data, perform portfolio analysis, and generate personalized reports. This enabled them to provide more tailored and timely financial advice to their clients, resulting in increased client satisfaction and improved business performance.
Lessons Learned
While each case study may have unique aspects, some common lessons can be derived from successful implementations of self-service analytics:
User-Centric Approach: Successful implementation of self-service analytics requires a user-centric approach. Understanding the needs and workflows of end-users is crucial for designing intuitive and user-friendly tools that align with their requirements.
Executive Support and Sponsorship: Executive support and sponsorship play a vital role in driving the adoption of self-service analytics. When leaders actively endorse and participate in the initiative, it creates a culture of data-driven decision-making and encourages wider adoption across the organization.
Training and Support: Providing comprehensive training programs and ongoing support is essential for the success of self-service analytics. Users need to be equipped with the necessary skills and knowledge to effectively use the tools and extract insights from data.
Data Governance and Security: Establishing robust data governance policies and security measures is crucial to maintain data integrity, protect sensitive information, and ensure compliance with regulations.
Continuous Improvement and Evaluation: Organizations should continuously monitor and evaluate the impact of self-service analytics. Gathering feedback, analyzing usage patterns, and identifying areas for improvement can help optimize the implementation and drive continuous innovation.
These case studies demonstrate the transformative power of self-service analytics in various industries and highlight the benefits organizations can achieve by empowering their business teams with data-driven insights. By learning from these examples and implementing best practices, organizations can unlock the full potential of self-service analytics and gain a competitive advantage in the marketplace.
Conclusion
Self-service analytics has emerged as a game-changer in the world of data analysis and decision-making. By empowering business teams with the ability to access, analyze, and derive insights from data on their own, organizations can accelerate the decision-making process, reduce dependence on IT departments, and foster a culture of data-driven collaboration.
In this blog post, we explored the concept of self-service analytics, its key features, and the benefits it brings to organizations. We discussed the importance of choosing the right tools, defining data governance policies, providing training and support, and encouraging collaboration and knowledge sharing.
We also addressed common challenges that organizations may face when implementing self-service analytics and provided solutions to overcome these obstacles. By addressing data quality and security concerns, ensuring user adoption and training, and addressing technical integration and resistance to change, organizations can successfully implement self-service analytics and reap its benefits.
Additionally, we examined real-world case studies that showcased the transformative impact of self-service analytics in organizations across various industries. These examples illustrated how self-service analytics empowered business teams, improved operational efficiency, and enhanced decision-making processes.
As organizations continue to navigate the data-driven landscape, self-service analytics will be a critical tool for unlocking the true potential of data and empowering business teams. By embracing self-service analytics, organizations can drive innovation, achieve better business outcomes, and gain a competitive edge in today's rapidly evolving business environment.
In conclusion, self-service analytics is an essential tool for empowering business teams, enabling faster decision-making, reducing reliance on IT, and fostering a culture of data-driven collaboration. By implementing self-service analytics with careful planning, training, and support, organizations can harness the power of their data and drive success in the digital age.